OCV Fitting#
Open-circuit voltage fitting is the key step for conducting Degradation Mode Analysis (DMA). PyProBE has a number of built-in methods for this.
[1]:
import pyprobe
import polars as pl
from pyprobe.analysis import degradation_mode_analysis as dma
import numpy as np
import matplotlib.pyplot as plt
2024-11-18 12:57:53,942 INFO util.py:154 -- Missing packages: ['ipywidgets']. Run `pip install -U ipywidgets`, then restart the notebook server for rich notebook output.
In this example, we are going to generate a synthetic aged OCV curve. We will use the half cell OCV fits from Chen 2020 [1].
[2]:
def graphite_LGM50_ocp_Chen2020(sto):
"""Chen2020 graphite ocp fit."""
u_eq = (
1.9793 * np.exp(-39.3631 * sto)
+ 0.2482
- 0.0909 * np.tanh(29.8538 * (sto - 0.1234))
- 0.04478 * np.tanh(14.9159 * (sto - 0.2769))
- 0.0205 * np.tanh(30.4444 * (sto - 0.6103))
)
return u_eq
def nmc_LGM50_ocp_Chen2020(sto):
"""Chen2020 nmc ocp fit."""
u_eq = (
-0.8090 * sto
+ 4.4875
- 0.0428 * np.tanh(18.5138 * (sto - 0.5542))
- 17.7326 * np.tanh(15.7890 * (sto - 0.3117))
+ 17.5842 * np.tanh(15.9308 * (sto - 0.3120))
)
return u_eq
z = np.linspace(0, 1, 1000) # stoichiometry vector
# generate complete ocp curves
ocp_pe = nmc_LGM50_ocp_Chen2020(z)
ocp_ne = graphite_LGM50_ocp_Chen2020(z)
We will now define a set of stoichiometry limits to generate our synthetic OCV curve:
[3]:
n_pts = 1000
# positive electrode
x_pe_lo = 0.85 # stoichiometry at low cell SOC
x_pe_hi = 0.27 # stoichiometry at high cell SOC
x_pe = np.linspace(x_pe_lo, x_pe_hi, n_pts) # stoichiometry range
# negative electrode
x_ne_lo = 0.03 # stoichiometry at low cell SOC
x_ne_hi = 0.9 # stoichiometry at high cell SOC
x_ne = np.linspace(x_ne_lo, x_ne_hi, n_pts) # stoichiometry range
# full cell voltage and capacity
voltage = nmc_LGM50_ocp_Chen2020(x_pe) - graphite_LGM50_ocp_Chen2020(x_ne)
capacity = np.linspace(0, 5, n_pts) # capacity range in Ah
plt.figure()
plt.plot(capacity, voltage)
plt.xlabel('Capacity (Ah)')
plt.ylabel('Voltage (V)')
[3]:
Text(0, 0.5, 'Voltage (V)')
We will now generate a fit to this voltage curve.
First, we must create instances of the OCP class to hold our electrode OCP information:
[4]:
neg_ocp = dma.OCP(graphite_LGM50_ocp_Chen2020)
pos_ocp = dma.OCP(nmc_LGM50_ocp_Chen2020)
In this example, we have directly declared the OCPs since we already have callable functions for them. Alternatively you can use the from_data
or from_expression
to define your OCP from data points or a Sympy expression.
Now we can fit to the ocv curve:
[5]:
# put the voltage and capacity data into a Result object (not necessary in normal use)
OCV_result = pyprobe.Result(base_dataframe=pl.DataFrame({'Capacity [Ah]': capacity, 'Voltage [V]': voltage}), info= {})
stoichiometry_limits, fitted_curve = dma.run_ocv_curve_fit(
input_data= OCV_result,
ocp_ne=neg_ocp,
ocp_pe=pos_ocp,
fitting_target='OCV',
optimizer='minimize',
optimizer_options={
'x0': np.array([0.9, 0.1, 0.1, 0.9]),
'bounds': [(0, 1), (0, 1), (0, 1), (0, 1)]
}
)
This produces two result objects. The first is the fitted stoichiometry limits:
[6]:
print(stoichiometry_limits.data)
shape: (1, 8)
┌────────────┬────────────┬────────────┬───────────┬───────────┬───────────┬───────────┬───────────┐
│ x_pe low ┆ x_pe high ┆ x_ne low ┆ x_ne high ┆ Cell ┆ Cathode ┆ Anode ┆ Li │
│ SOC ┆ SOC ┆ SOC ┆ SOC ┆ Capacity ┆ Capacity ┆ Capacity ┆ Inventory │
│ --- ┆ --- ┆ --- ┆ --- ┆ [Ah] ┆ [Ah] ┆ [Ah] ┆ [Ah] │
│ f64 ┆ f64 ┆ f64 ┆ f64 ┆ --- ┆ --- ┆ --- ┆ --- │
│ ┆ ┆ ┆ ┆ f64 ┆ f64 ┆ f64 ┆ f64 │
╞════════════╪════════════╪════════════╪═══════════╪═══════════╪═══════════╪═══════════╪═══════════╡
│ 0.85 ┆ 0.27 ┆ 0.03 ┆ 0.9 ┆ 5.0 ┆ 8.62069 ┆ 5.747127 ┆ 7.5 │
└────────────┴────────────┴────────────┴───────────┴───────────┴───────────┴───────────┴───────────┘
And the second is a result object containing the fitted OCP curve:
[7]:
fig = pyprobe.Plot()
fig.add_line(fitted_curve, x='SOC', y='Input Voltage [V]',label = "Input")
fig.add_line(fitted_curve, x='SOC', y='Fitted Voltage [V]', color='red', label = 'Fit', dash='dash')
fig.show_image()
# fig.show() # This will show the plot interactively, it is commented out for the sake of the documentation
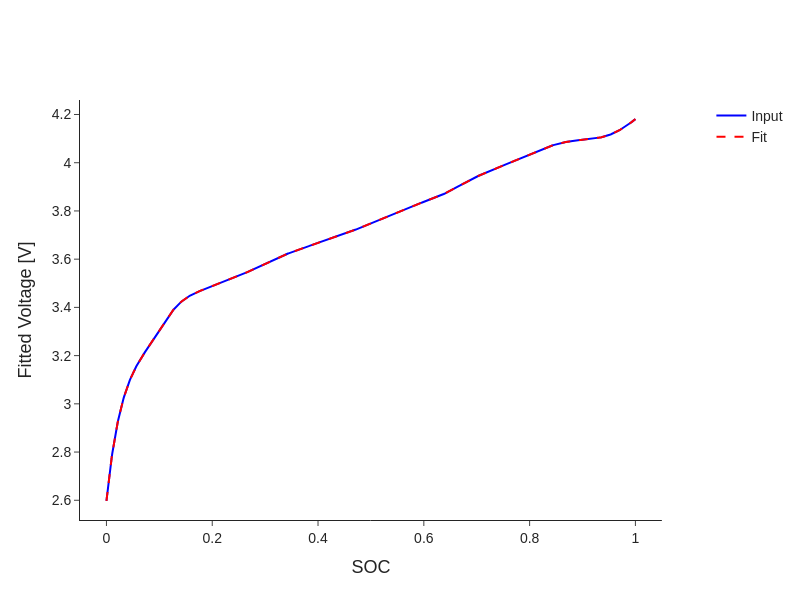
You can also fit to differentiated voltage data:
[8]:
stoichiometry_limits, fitted_curve = dma.run_ocv_curve_fit(
input_data= OCV_result,
ocp_ne=neg_ocp,
ocp_pe=pos_ocp,
fitting_target='dQdV',
optimizer='differential_evolution',
optimizer_options={
'bounds': [(0.8, 1), (0.2, 0.3), (0, 0.1), (0.86, 1)]
}
)
print(stoichiometry_limits.data)
fig = pyprobe.Plot()
fig.add_line(fitted_curve, x='SOC', y='Input dSOCdV [1/V]',label = "Input")
fig.add_line(fitted_curve, x='SOC', y='Fitted dSOCdV [1/V]', color='red', label = 'Fit', dash='dash')
fig.show_image()
# fig.show() # This will show the plot interactively, it is commented out for the sake of the documentation
shape: (1, 8)
┌────────────┬────────────┬────────────┬───────────┬───────────┬───────────┬───────────┬───────────┐
│ x_pe low ┆ x_pe high ┆ x_ne low ┆ x_ne high ┆ Cell ┆ Cathode ┆ Anode ┆ Li │
│ SOC ┆ SOC ┆ SOC ┆ SOC ┆ Capacity ┆ Capacity ┆ Capacity ┆ Inventory │
│ --- ┆ --- ┆ --- ┆ --- ┆ [Ah] ┆ [Ah] ┆ [Ah] ┆ [Ah] │
│ f64 ┆ f64 ┆ f64 ┆ f64 ┆ --- ┆ --- ┆ --- ┆ --- │
│ ┆ ┆ ┆ ┆ f64 ┆ f64 ┆ f64 ┆ f64 │
╞════════════╪════════════╪════════════╪═══════════╪═══════════╪═══════════╪═══════════╪═══════════╡
│ 0.849925 ┆ 0.27001 ┆ 0.030007 ┆ 0.900009 ┆ 5.0 ┆ 8.62196 ┆ 5.747114 ┆ 7.500472 │
└────────────┴────────────┴────────────┴───────────┴───────────┴───────────┴───────────┴───────────┘
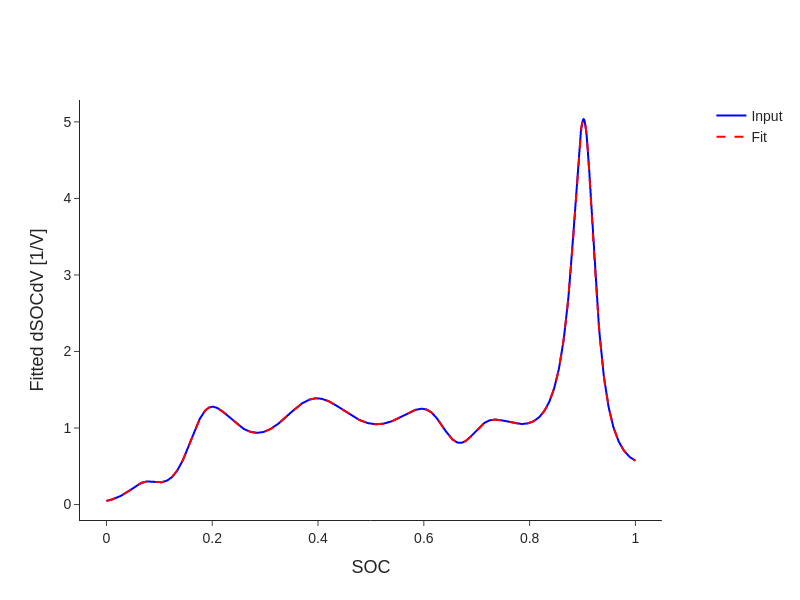
Chen CH, Planella FB, O’Regan K, Gastol D, Widanage WD, Kendrick E. Development of Experimental Techniques for Parameterization of Multi-scale Lithium-ion Battery Models. Journal of The Electrochemical Society. 2020;167(8): 080534. https://doi.org/10.1149/1945-7111/AB9050.